My PhD thesis
Variational methods for model-based image segmentation
This thesis has been realized from 2010 to 2013, in the context of an industrial collaboration with Philips Research (Paris, France). It has been awarded the 2014 Thesis Prize by the Dauphine Foundation and the AMIES Award for mathematics applied to industrial problems.
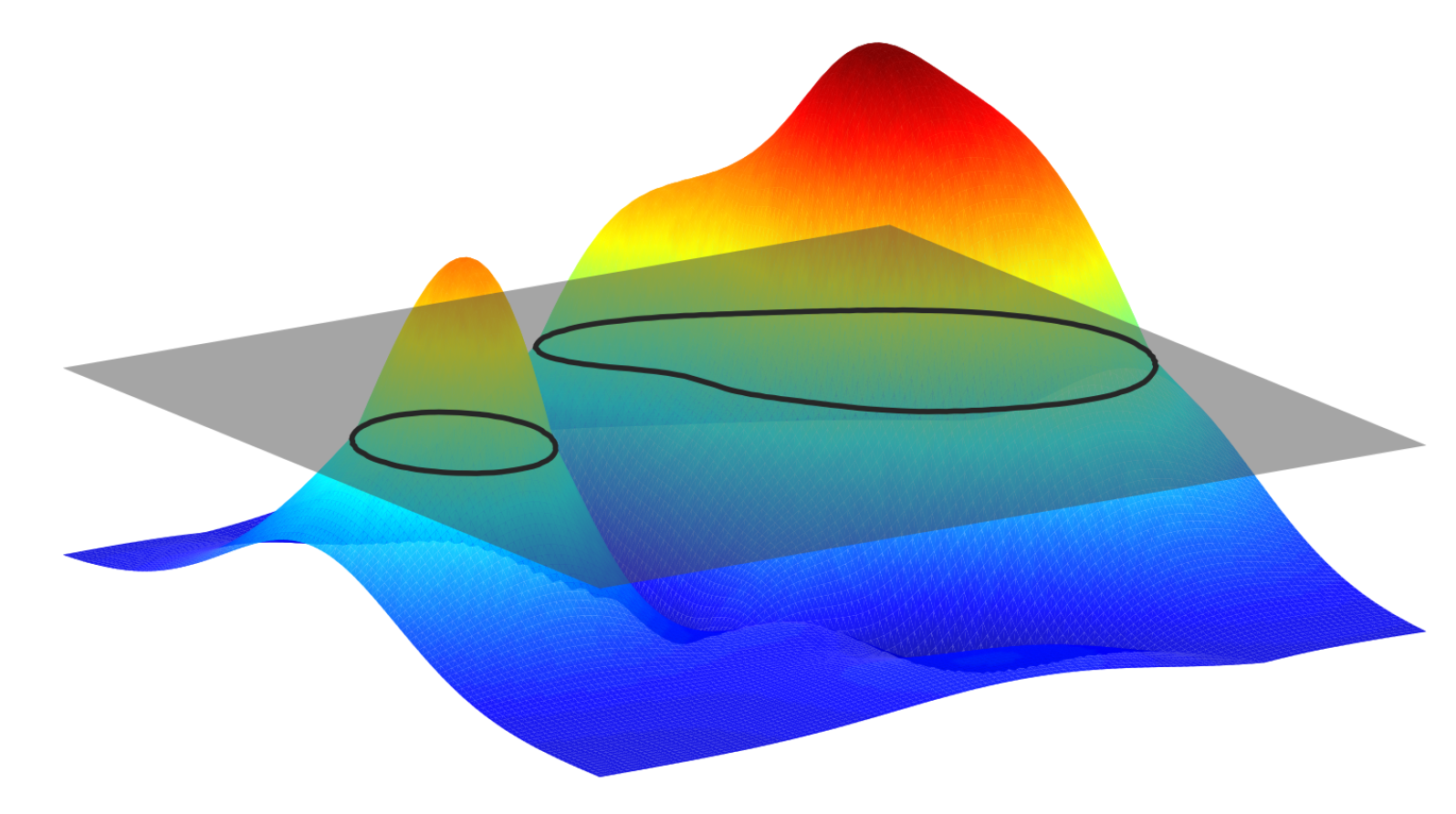
Variational methods for model-based image segmentation - Applications in medical imaging
Download PDF Download Slides
http://tel.archives-ouvertes.fr/tel-00932995
Within the wide field of medical imaging research, image segmentation is one of the earliest topics but still active. Retrieving the shape of an organ is indeed of high interest for diagnosis, therapy planning or medical research in general. While automated methods are desirable for the sake of reproducibility, they are difficult to design in a generic and robust way. This thesis focuses on model-based methods, which achieve a good trade-off between customization (models carry prior knowledge on the target structure) and genericity (which is important for research efficiency).
The goal of this thesis is to build an efficient segmentation framework that is able to leverage all kinds of external information, i.e. pre-segmented databases via statistical learning, other images from the same patient via co-segmentation and user input via live interactions with the algorithm. This work is based on the implicit template deformation framework, which is a variational method relying on an implicit representation of shapes. After improving the mathematical formulation and the optimization process of this approach, we show its potential on challenging clinical problems. Then, we introduce throughout the remainder of the thesis different generalizations aimed at enriching the model. All the presented extensions are independent but complementary. This thesis therefore eventually transforms the implicit template deformation algorithm into a powerful segmentation framework that is able to (i) learn from a database useful information about both the shape and appearance of the target organ, (ii) use simultaneously multiple images from the same patient. Special care is taken to preserve the most interesting properties of template deformation such as computational efficiency, topology preservation and the ability to take into account user interactions.
The diversity of the clinical applications addressed across this thesis (such as kidney segmentation in ultrasound, contrast-enhanced ultrasound and CT 3D images or myocardium segmentation in ultrasound and MR images) shows the genericity and the effectiveness of the proposed contributions.
Keywords image segmentation
, medical imaging
, model-based methods
, variational methods
, statistical learning
, kidney
, ultrasound
, contrast-enhanced ultrasound
Jury
President | Nicholas Ayache | INRIA Sophia-Antipolis |
Reviewer | Daniel Rueckert | Imperial College London |
Reviewer | Anthony Yezzi | Georgia Institute of Technology |
Examiner | Roberto Ardon | Philips Research Medisys |
Examiner | Gabriel Peyre | Universite Paris Dauphine |
Advisor | Laurent D. Cohen | Universite Paris Dauphine |